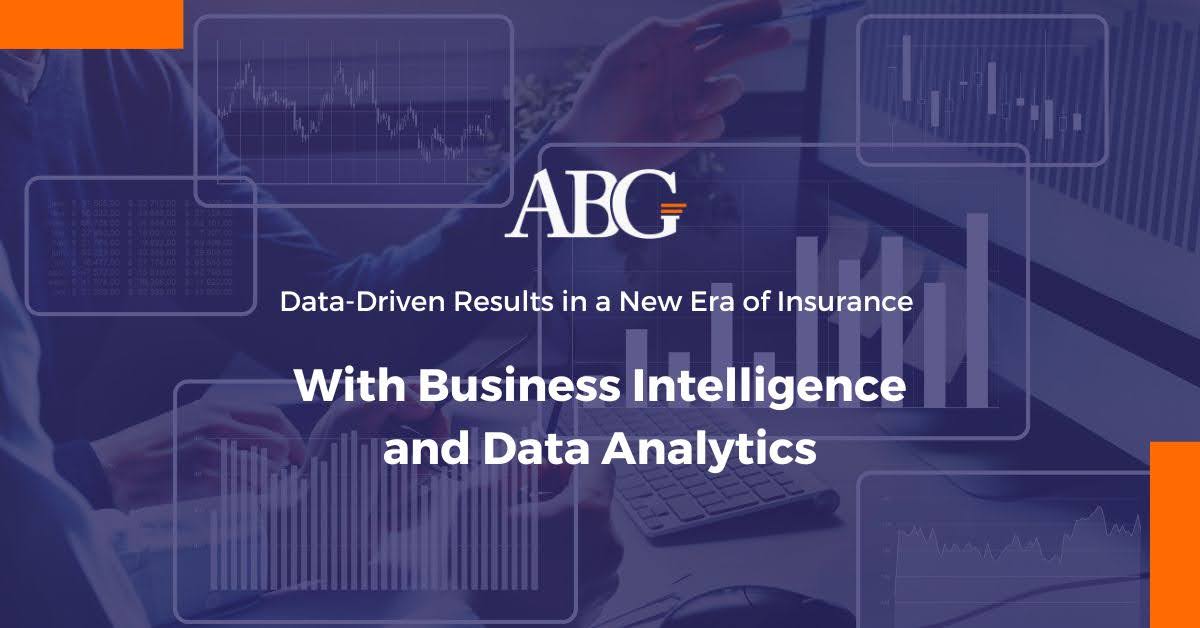
The banking industry is reeling from the effects of fraud. In 2017, a total of US$1.7 billion was lost to bank fraud, a 7% increase from 2016. This is not only a problem for banks but also for customers who are often left with the consequences of this type of crime.
Banks are always on the lookout for new ways to detect fraud and this is where machine learning comes in handy. Machine learning is a type of artificial intelligence that can be used to find patterns in data.
Machine learning algorithms are used by banks to learn from past patterns and identify new fraud cases. They help banks detect unusual transactions and flag them as potential frauds, thus saving the bank from losses.
Machine learning and data analysis can be used to detect fraudulent activity in real time and reduce the impact on customers. Banks are increasingly turning to machine learning methods as they are more accurate than other methods, such as human analysts or rules-based algorithms.
Measures for Bank Frauds prevention and detection
Bank frauds are a growing concern in the financial sector. They can be prevented by implementing different measures to monitor and detect them.
Some of these measures are:
– Implementing a system that can identify suspicious transactions and prevent fraud before it happens
– Using card readers with encryption technology to avoid data breaches
– Monitoring ATM activity to detect any suspicious behavior
ABG’s Fraud Detection Systems Used by Banks
With ABG’s AI, ML, and regtech technologies, your financial institution will be:
Equipped with optimum fraud detection, effective investigations, aggregated suspicious activity monitoring, and reporting will take a proactive protection approach.
Apply an advanced strategy for detecting suspicious transactions in areas such as AML, CFT sanctions screening, and beneficial ownership.
Use hybrid analytics to handle alerts, test scenarios, respond faster to evolving risks, and comply with banking regulations.
Find fraud and reduce false positives by processing all data in real-time or batch.